Let's Master AI Together!
Refining Recommendations with User Feedback Loops
Written by: Chris Porter / AIwithChris
The Power of User Feedback Loops in Recommendation Systems
Feedback is an essential component of any recommendation system. While algorithms can analyze vast amounts of data, the true magic happens when these systems are designed to learn from user feedback. User feedback loops serve as a bridge between technology and human behavior, allowing systems to adapt and refine their recommendations continuously. This sets the stage for a more personalized experience that keeps users engaged and satisfied.
User feedback loops take many forms, from explicit feedback—such as ratings and reviews—to implicit feedback, such as user interactions and dwell time on content. Interestingly, user behavior often reflects unexpressed preferences, which can provide invaluable insights for refining recommendation algorithms. Harnessing this data effectively can lead to a comprehensive understanding of user needs and preferences.
When companies integrate user feedback into their recommendation systems, they not only enhance the personalization of their offerings but also build a community of engaged users. The adaptive nature of these systems fosters loyalty and encourages greater user interaction. Moreover, continuous refinement of the algorithms based on real-time user feedback allows organizations to stay ahead of changing preferences, ensuring that their suggestions remain relevant.
Utilizing user feedback effectively requires a well-thought-out strategy. Organizations need to define what types of feedback they will solicit, how they will gather it, and the methods to incorporate it into their existing systems. For example, engaging users through surveys, A/B testing, or monitoring behavioral data can provide tailored insights that refine recommendation algorithms. The challenge lies in distinguishing between noise and genuine insights, which necessitates careful analysis and real-time adjustments. With an effective feedback loop established, organizations can create a cycle of improvement driven by user preferences.
Understanding Different Types of User Feedback
Diving deeper into user feedback loops, it's essential to categorize the types of feedback that can be collected. These fall broadly into two categories: explicit and implicit feedback. Explicit feedback involves direct user input, such as ratings or reviews, where users convey their opinions about specific items or content. This kind of feedback is often straightforward for algorithm developers to understand and utilize, leading to immediate adjustments in recommendations.
On the other hand, implicit feedback is subtler and often takes the shape of user behavior. This can include actions such as clicking on an item, time spent on a page, or frequently revisiting specific content. Analyzing this data can provide a clearer picture of users' likes and dislikes without them having to articulate it directly. By leveraging both types of feedback, organizations can create a 360-degree view of user preferences, enhancing the overall recommendation experience.
Moreover, collecting user feedback should not be a one-time event but should instead be an ongoing process. Regularly soliciting user input through periodic surveys or feedback prompts can help keep the recommendation system aligned with current user needs. This steady stream of information allows organizations to respond swiftly to shifts in user sentiment or preferences, ensuring that their recommendations remain timely and relevant.
Utilizing robust data collection methods helps organizations gather the feedback they need to iterate on their recommendations. From sophisticated analytics tools to simple rating systems, the right technology can help capture and interpret user feedback, paving the way for smarter recommendations. Employing machine learning algorithms capable of analyzing this data in real time empowers organizations to respond dynamically to user needs.
Challenges and Strategies for Implementing Feedback Loops
Despite the benefits of user feedback loops, organizations often face numerous challenges when integrating them into their recommendation systems. One significant hurdle is data quality. Organizations must ensure they are collecting accurate feedback that reflects genuine user sentiments. Inconsistent feedback can skew results and, subsequently, the recommendations users receive.
Another challenge lies in user engagement. If users do not feel motivated or see the value in providing feedback, the loop may become stagnant, undermining the entire process. To combat this, organizations should consider implementing incentives for users to provide feedback, such as discounts, rewards, or even gamification elements. This can encourage higher participation rates and yield higher quality insights.
In addition to engaging users, it's crucial for organizations to create feedback loops that are easy to navigate. A cumbersome or complicated feedback process can discourage users from participating, leading to incomplete data collection. By streamlining this process and making it intuitive, organizations can enhance the likelihood of receiving valuable feedback.
Understanding how to best interpret and act on this feedback is also vital. Collecting feedback is just the first step; organizations must be prepared to analyze it and translate it into actionable insights. This often requires sophisticated data analytics capabilities and a team skilled in interpreting complex data sets. By investing in these areas, organizations can ensure their feedback loops are working effectively, driving continual improvement in their recommendations.
Measuring the Effectiveness of Feedback Loops
Knowing whether user feedback loops are yielding the desired results is crucial for organizations. Measuring their effectiveness involves looking at key performance indicators (KPIs) that can indicate not only user satisfaction but also engagement levels. Common metrics include click-through rates on personalized recommendations, user retention rates, and conversion rates from recommendations.
In addition, qualitative metrics such as user sentiment, often gathered through surveys or social media monitoring, can provide deeper insights into how well feedback loops are functioning. Understanding whether users feel more satisfied and engaged with the recommendations can illuminate the overall success of the feedback mechanism.
Moreover, it’s essential for organizations to adapt their KPIs for different types of feedback. For example, explicit feedback metrics might focus more on accuracy and relevance, while implicit feedback metrics might analyze engagement and retention. By using a combination of both sets of metrics, organizations can create a more nuanced understanding of their user experience, allowing them to refine their recommendations continuously.
Regularly assessing the feedback loop's performance is imperative for growth. Organizations can establish a review system that matches the cadence of the feedback collection process. This could range from monthly reviews to quarterly assessments, depending on the organization’s pace of change. Such regular evaluation highlights areas for improvement, ensuring that user preferences are consistently respected.
Maintaining User Trust Through Transparent Processes
Transparency in how user feedback is collected and utilized plays a crucial role in fostering trust between organizations and their users. When users understand how their feedback influences recommendations, they are more likely to participate engage with the system. Companies should be open about their processes and let users know how their input is being translated into enhanced recommendations.
Additionally, maintaining user anonymity and data privacy is vital. Users need assurance that their feedback will not be misused or compromise their privacy. Organizations must adhere to strict data protection standards and policies, ensuring that user feedback loops are not only effective but also ethical.
Engagement strategies can also contribute to transparency. Keeping users informed of changes made to recommendation algorithms based on their feedback can establish a feedback culture characterized by open communication. By creating forums or feedback channels where users can express their thoughts and see the impact of their feedback, organizations can cultivate a more trusting community.
Conclusion: The Future of Personalized Recommendations
Refining recommendations through user feedback loops is a fundamental element in crafting a personalized user experience. As user expectations evolve and technology continues to advance, organizations must embrace feedback systems that adapt proactively to changing preferences. The ongoing integration of explicit and implicit feedback creates a dynamic environment rich with opportunities for growth and innovation.
Organizations capable of harnessing user feedback effectively position themselves to foster user loyalty, enhance engagement, and drive conversions. Learning how to blend technology with user insights defines the future of recommendation systems. Organizations that prioritize continuous improvement through user feedback will undoubtedly stand out in a crowded digital landscape.
If you're eager to learn more about AI and how to leverage user feedback loops for refining recommendations, visit AIwithChris.com for a wealth of resources and insights to guide you on your journey.
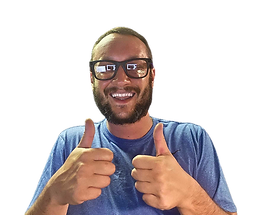_edited.png)
🔥 Ready to dive into AI and automation? Start learning today at AIwithChris.com! 🚀Join my community for FREE and get access to exclusive AI tools and learning modules – let's unlock the power of AI together!