Let's Master AI Together!
Learning Intuitive Physics: Advancing AI Through Predictive Representation Models
Written by: Chris Porter / AIwithChris
The Emergence of Intuitive Physics in AI

*Image Source: MarkTechPost*
Artificial Intelligence has made significant strides in various fields, and one of the most intriguing areas of study is intuitive physics. This concept revolves around teaching machines to grasp and predict physical phenomena in a manner similar to human cognition, without explicit programming. The progress in this field has been fueled by recent innovations in predictive representation models, allowing AI to comprehend and simulate complex physical interactions.
The growing demand for AI systems to operate in diverse environments has led researchers to develop frameworks capable of learning from a plethora of data sources. As the technology matures, applications in autonomous vehicles, robotics, and virtual simulations become increasingly viable, showcasing the importance of intuitive physics in the advancement of AI. A prominent example of this advancement is the 3D-IntPhys framework, which is designed to facilitate a more profound understanding of three-dimensional environments.
3D-IntPhys Framework: Redefining Predictive Modeling
The 3D-IntPhys Framework represents a significant leap in AI's ability to learn 3D-grounded visual intuitive physics models. This is accomplished through the analysis of videos depicting complex scenes. Unlike traditional models, which rely heavily on predefined rules and simulations, 3D-IntPhys extracts essential information directly from raw images, enabling it to make long-horizon future predictions effectively.
One of the standout features of this framework is its capability to create accurate predictions over extended timeframes by understanding the dynamics within a scene. For instance, given a video of a ball rolling down a hill, the framework can predict the trajectory and final position of the ball, even when faced with new situations it hasn’t encountered before. This ability is crucial for applications such as robotics and athletic performance analysis, where understanding physical interactions can lead to better designs and training methodologies.
Moreover, the 3D-IntPhys framework is engineered to demonstrate strong generalization in complex scenarios, especially under extrapolation settings. This means that it can apply learned knowledge to unfamiliar environments and predict outcomes accurately, which is essential for introducing AI solutions into real-world applications. By moving beyond 2D representations, this technology opens up numerous possibilities for realistic simulations and autonomous decision-making tasks.
AI Enhancements in Physics Simulation
Traditional physics simulations often require significant computation and detailed programming, but AI introduces an innovative solution to this challenge. By leveraging vast datasets of prior simulations, AI models can learn to approximate physical phenomena with far less computational effort. This efficiency not only speeds up the simulation process but also allows for more complex scenarios to be analyzed in real-time.
The integration of AI into physics simulations enhances traditional methods by identifying knowledge gaps and filling them based on learned data. For example, machine learning algorithms can sift through historical simulation data to recognize patterns and predict system behaviors that existing models might overlook. This uniqueness allows for quicker adaptations and improvements in fields such as fluid dynamics, structural analysis, and even social dynamics.
AI models are notably equipped to handle large-scale simulations, thanks to their ability to parse through immense amounts of data efficiently. This capability drastically reduces the time and resources typically required for investigating complex physical interactions, paving the way for advancements in engineering and scientific research that were once unattainable.
Intuitive Physics Understanding Through Multimodal Learning
Another exciting aspect of learning intuitive physics with AI involves the use of multimodal large-language models and video prediction methods. Recent research shows that self-supervised training can enable models to develop a robust understanding of intuitive physics. The architecture designed for this purpose, known as V-JEPA, learns abstract representations that prioritize predictable information while disregarding lower-level features that do not contribute to understanding physical behaviors.
The effectiveness of V-JEPA is highlighted by its ability to outperform untrained networks, particularly in scenarios involving impossible actions or unlikely physical interactions. This is a game-changer for industries relying on predictive AI, as it showcases the potential of large-language models in driving advancements in intuitive physics understanding. Not only does this approach present AI with more nuanced data, but it also equips it to recognize contradictions and anomalies within physical interactions, establishing a clearer comprehension of real-world physics.
Through extensive training on diverse datasets, AI systems can become adept at simulating physical events and understanding the underlying laws governing motion, interaction, and transformation. This capability opens avenues for more sophisticated applications in augmented reality, educational technology, and interactive gaming—all areas that stand to benefit from AI's enhanced understanding of physical principles.
AI and Physics Modeling: Accuracy Meeting Efficiency
The application of AI in physics modeling is an emerging field with transformative potential. Currently, AI methodologies, particularly neural networks and machine learning, are being employed to study and predict a wide array of physical processes. The promise of these technologies lies in their ability to learn directly from empirical or simulation data, providing results that can be both accurate and swift compared to traditional physics models.
However, the success of AI-centric approaches is contingent upon the quality and richness of the data available. High-quality datasets yield better modeling outcomes, making the integration of data collection techniques paramount for building robust AI paradigms. Issues can arise when dealing with underrepresented phenomena or unexpected environmental conditions, which may introduce additional complexity in predictions. This intricacy highlights the need for continuous refinement and validation of models against experimental results.
Leveraging AI to enhance our understanding of physics is especially beneficial in industries such as aerospace, automotive, and energy, where predicting complex interactions can translate to savings in both cost and time. For example, an autonomous vehicle utilizing AI-driven models can predict not only its current trajectory but also future interactions with other objects in dynamic environments, ensuring safer navigation.
The need for accurate and efficient physics modeling has never been greater, as technology continuously advances and systems grow more interconnected. The evolution of AI methods not only aids in addressing complex problems but also assists researchers and engineers in devising innovative solutions that were once inconceivable.
Conclusion: The Future of AI in Physics
As the field of AI evolves, the focus continues to shift towards mastering intuitive physics through predictive representation models. Tools like the 3D-IntPhys framework and methodologies that enhance traditional simulations are reshaping how we develop and interact with AI technologies. The journey towards sophisticated AI systems capable of comprehending and predicting physical phenomena offers vast potential for numerous sectors.
Whether in robotics, autonomous systems, or virtual modeling, the implications of mastering intuitive physics are far-reaching. Those interested in delving deeper into these advancements are encouraged to explore this fascinating intersection of AI and physics. By harnessing these cutting-edge developments, we can unlock untapped potential and rewrite the future of technology.
For more insightful information on AI and its advancements, check out AIwithChris.com for comprehensive resources, articles, and research.
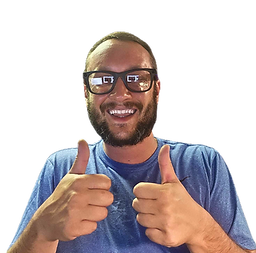_edited.png)
🔥 Ready to dive into AI and automation? Start learning today at AIwithChris.com! 🚀Join my community for FREE and get access to exclusive AI tools and learning modules – let's unlock the power of AI together!