Let's Master AI Together!
Building Trust with Conversational AI: How to Avoid Common Pitfalls
Written by: Chris Porter / AIwithChris
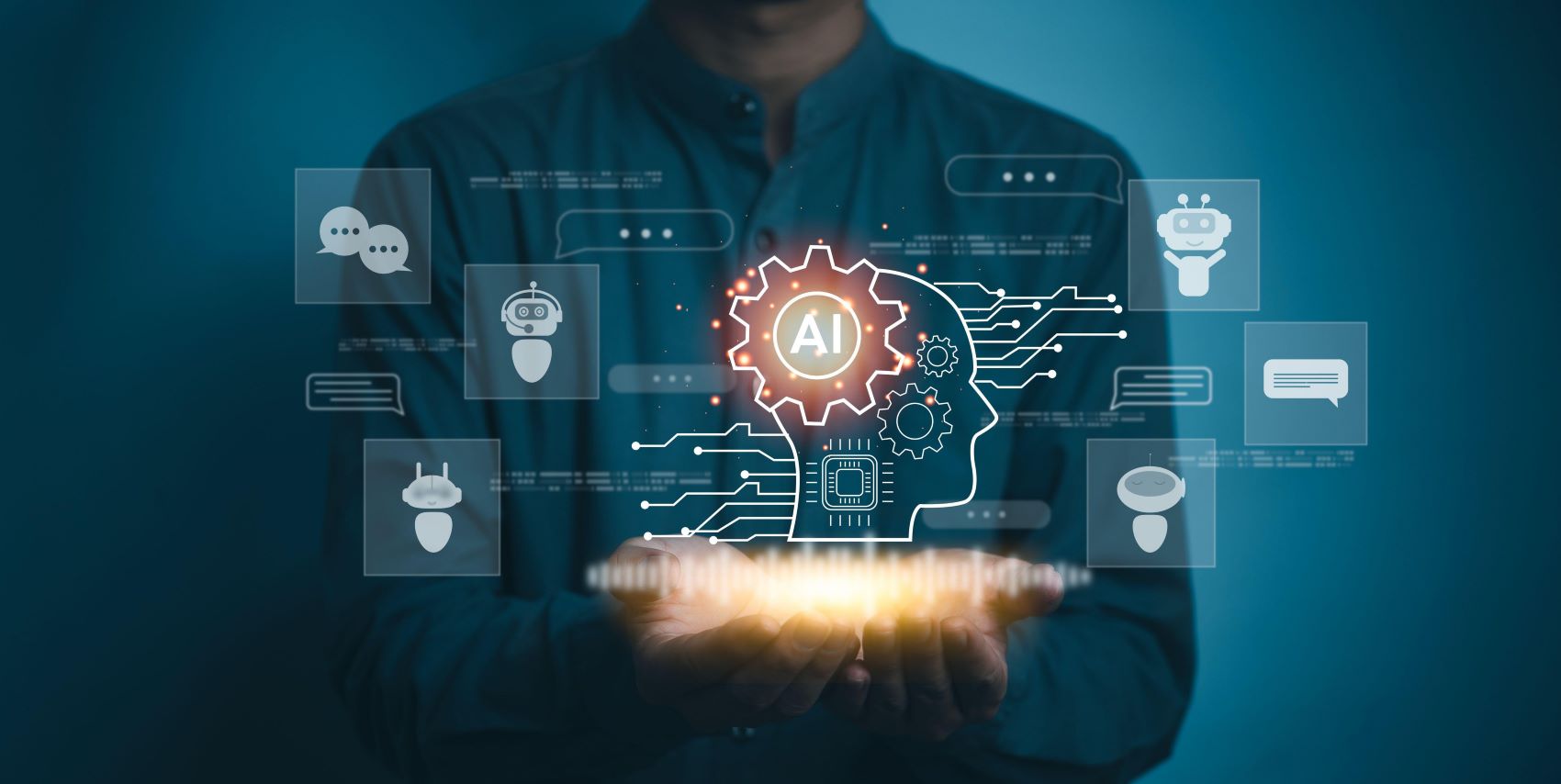
Image Source: Alamy
Fostering Confidence in AI Interactions
As technology continues to advance, the integration of Conversational AI in various sectors has skyrocketed. From customer service to healthcare, the ability to interact with clients and patients through AI has transformed service delivery. However, building trust in these AI systems is paramount for their successful deployment. Without trust, users may be hesitant to engage fully, diminishing the potential benefits. Here’s a closer look at some common pitfalls and how organizations can avoid them while fostering trust in Conversational AI.
Involvement of Stakeholders
The design and implementation of Conversational AI tools should not occur in isolation. In fact, involving multiple stakeholders is crucial in building a robust framework that aligns with the organization's values. When diverse perspectives are integrated into the design process, the result is a more nuanced conversational AI that resonates with users. Common stakeholders may include end-users, developers, business leaders, and legal advisors, each bringing valuable insights that shape user interaction and experience. Feedback from stakeholder engagement also aids in refining the AI’s responses and ensuring cultural and ethical considerations are met, further establishing trust. This collective input can prevent misalignment between user expectations and the AI's outputs, thereby minimizing potential miscommunication.
In addition to improving alignment with organizational values, involving stakeholders enhances the ethical considerations of Conversational AI. When various voices contribute, the AI can better navigate complex situations, especially in sensitive fields such as healthcare and legal services. For instance, stakeholders can help identify scenarios where AI might misinterpret user intent or provide misleading information, equipping organizations to address these vulnerabilities effectively.
Ethical Considerations
Ethical concerns are a recurring theme in discussions around Conversational AI, particularly in high-stakes sectors. The legal industry, for instance, presents unique challenges, as the unsupervised use of AI can lead to severe professional responsibility issues. AI systems programmed to provide legal advice may inadvertently dispense incorrect guidance, leading to potential malpractice. This underlines the importance of ethical considerations, not just for compliance, but to uphold the integrity of these essential services.
Organizations must navigate these robotic pitfalls thoughtfully to ensure Conversational AI cannot only serve its intended purpose but also support ethical practice. One strategy involves clear guidelines regarding the scope of the AI's functionality. Limitations must be set, defining what is appropriate for AI to handle while equipping users with knowledge about when to seek human intervention. Additionally, regularly auditing AI interactions can identify any patterns or errors requiring immediate attention. Transparency surrounding these issues is critical; organizations should communicate openly with users about the capabilities and limitations of their AI systems.
Ensuring Explainability and Transparency
High levels of explainability and transparency in Conversational AI are necessary for user trust. When users understand how the AI reaches its conclusions and its underlying logic, they are more likely to embrace the technology. To achieve this, organizations can leverage Knowledge Graphs in tandem with Large Language Models (LLMs), enhancing the transparency of the AI’s decisions while safeguarding data security.
Integrating Knowledge Graphs aids in creating context-aware conversations, as they provide necessary background information to the AI, leading to more meaningful interactions. By making the inner workings of the AI open and comprehensible, organizations can empower users to dissect the rationale behind recommendations, thus reinforcing their trust in the system. Coupled with effective training that educates users on interpreting AI outputs, this measure bolsters confidence, transforming users from passive consumers to informed participants in the interaction.
Data Privacy and Security Measures
With the increasing prevalence of data breaches and privacy violations, ensuring strict data privacy and security protocols is essential for any Conversational AI system. Security measures must maintain user confidentiality while ensuring compliance with laws and regulations, such as GDPR or HIPAA, depending on the sector. Implementing Role-Based Access Control (RBAC) is a fundamental approach, aligning access levels with organizational policies. By granting access only to authorized personnel, organizations can mitigate the risks associated with unauthorized data exposure.
Data encryption is another critical aspect of ensuring user safety, as it protects sensitive information during transmission. Organizations must employ encryption both at rest and in transit, guaranteeing that no unauthorized parties intercept or manipulate data. Regular security assessments and vulnerability scans also play a vital role in identifying and mitigating risks before they lead to potential breaches. Combined, these security measures establish a solid foundation for building user trust in AI systems, as users will feel secure knowing their data is treated with the utmost respect and diligence.
The Need for Human Oversight
Even though Conversational AI systems can process vast amounts of interactions with users, human oversight remains essential to uphold quality control. Automated systems, while efficient, must be monitored to prevent potential mistakes, miscommunications, or biases affecting user interactions. By having trained personnel monitor AI activities, organizations can quickly identify areas of concern and take corrective actions.
Human oversight should involve regularly reviewing AI interactions and determining whether the system adheres to the defined parameters. When discrepancies are found, investigations can take place to ascertain whether the AI requires adjustments or the guidelines need refinement. Thus, organizations can ensure AI behaves appropriately, fostering user trust and long-term engagement.
Building a Trustworthy AI Ecosystem
Creating a trustworthy and reliable Conversational AI system combines the aforementioned elements into a coherent strategy. Organizations must commit to continuous improvement, regularly solicit feedback, and address ethical considerations head-on. Open communication about AI capabilities, involvement of stakeholders in the design process, and maintaining high standards for data privacy will not only bolster user confidence but also pave the way for successful AI adoption. A trustworthy AI ecosystem is a collaborative effort; as AI technologies evolve, organizations must adapt and refine approaches to ensure that users feel secure and valued at every juncture.
Conclusion
Building trust in Conversational AI is a multifaceted endeavor, but when stakeholders are involved, ethical considerations are prioritized, and systems operate with transparency, it leads to a successful integration of AI solutions across various industries. Organizations can cultivate lasting relationships with users by ensuring their voice is heard, their data is protected, and they understand the technology at play. For further insights and guidance on how to maximize the advantages of AI in your organization, visit AIwithChris.com, where the latest tips and strategies await.
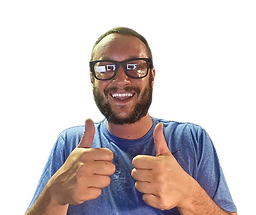_edited.png)
🔥 Ready to dive into AI and automation? Start learning today at AIwithChris.com! 🚀Join my community for FREE and get access to exclusive AI tools and learning modules – let's unlock the power of AI together!