Let's Master AI Together!
10 New AI Challenges—and How to Meet Them
Written by: Chris Porter / AIwithChris

Image source: Foreign Policy
Navigating the Evolving Landscape of AI
The rise of artificial intelligence (AI) within various sectors has ushered in numerous opportunities and advantages. With powerful algorithms and vast amounts of data, organizations can derive insights, automate processes, and enhance decision-making. However, the journey towards seamless AI adoption is not without its challenges. In this article, we will delve into ten new AI challenges organizations face today and explore actionable strategies for overcoming them.
1. Data Quality and Availability
Data is the lifeblood of AI, and its quality directly influences the efficacy of AI implementations. A significant challenge arises when organizations must ensure access to accurate, comprehensive, and unbiased data. Poor data quality can lead to incorrect predictions and flawed outcomes, so investing in a robust data governance framework is vital.
Organizations should prioritize data cleansing processes to remove inconsistencies and inaccuracies. They can also harness data augmentation techniques to enhance their existing datasets and ensure diversity in training data. Furthermore, regular audits and updates are essential for maintaining data integrity over time. Implementing real-time data monitoring tools can help track anomalies and facilitate quick corrective actions when needed.
Accessibility is another element of data quality that organizations need to tackle. In many cases, data silos exist where valuable information resides in different departments, leading to fragmented insights. To combat this, companies should adopt centralized data management systems that unify data from various sources. By breaking down these silos, organizations can leverage a comprehensive dataset that enhances the AI’s learning capabilities.
2. Algorithmic Bias
Another pressing challenge in AI development is algorithmic bias. AI systems can perpetuate existing biases found in training data, resulting in unfair or discriminatory outcomes. This bias can stem from historical prejudices embedded in the data or from the choices made during data collection.
To mitigate algorithmic bias, organizations need to implement fairness checks as part of the AI development lifecycle. This could involve employing bias detection tools that evaluate the training dataset for imbalance or discriminatory patterns. Diverse teams should be involved in the AI development process to provide various perspectives and potential solutions that address these biases head-on.
Moreover, organizations must establish a corrective framework that allows for re-evaluation and adjustment of biased algorithms. This involves continuous monitoring and periodic assessments of the AI’s outputs against predefined fairness criteria. Transparency in the outcomes generated by AI systems can also help maintain accountability and trust among users.
3. Explainability and Transparency
AI's “black box” nature presents another hurdle. Stakeholders often struggle to understand how complex algorithms make decisions, leading to skepticism and distrust. This lack of explainability can hinder the deployment of AI solutions, particularly in industries like healthcare and finance, where accountability is essential.
To address this challenge, organizations are increasingly adopting explainable AI (XAI) techniques. XAI focuses on developing models that can provide clear and interpretable explanations for their decisions. For instance, implementing models based on rule-based systems or using techniques such as LIME (Local Interpretable Model-agnostic Explanations) can provide insights into how specific inputs influence outputs.
Enhancing transparency is also key. Organizations should ensure that stakeholders have access to information on how algorithms are developed, trained, and tested. Regular communication about the measures taken to ensure representativeness in data can facilitate a better understanding of AI decisions, ultimately fostering trust.
4. Data Privacy and Security
With the rise of AI technology, concerns about data privacy and security have intensified. AI systems often require access to vast amounts of personal or sensitive information, making it critical for organizations to handle this data responsibly. Breaches can lead to not only financial damages but also a loss of trust from users.
Organizations must adopt stringent data protection measures compliant with regulations such as GDPR (General Data Protection Regulation) or CCPA (California Consumer Privacy Act). This includes implementing data encryption, conducting vulnerability assessments, and securing access controls to sensitive data. Moreover, fostering a culture of data privacy awareness training among employees can reduce the risk of human errors leading to breaches.
Regular audits and a commitment to transparency about how user information is managed can further bolster data security. By maintaining a proactive stance towards data privacy, organizations can ensure their AI implementations gain user trust and adhere to ethical standards.
5. Regulatory Compliance
As regulatory frameworks surrounding AI technologies evolve, organizations must remain vigilant in staying informed about the laws and ethical standards that govern their AI applications. The challenge lies in the differing standards across jurisdictions and industries, which can complicate compliance efforts.
To navigate this landscape effectively, organizations should establish dedicated teams responsible for monitoring regulations and ensuring compliance. Utilizing technology to streamline compliance processes can also enhance efficiency. For instance, organizations can leverage AI tools to automate tracking regulatory updates, flagging relevant changes that may impact AI operations.
Engaging in industry groups or forums focused on AI regulations can provide organizations with valuable insights and best practices for compliance. By proactively addressing these regulatory challenges, companies can mitigate risks and foster a culture of ethical AI development.
6. Integration with Existing Systems
Organizations often face challenges integrating AI technologies into their existing systems and processes. A poorly managed integration can lead to inefficiencies, wasted resources, and suboptimal performance of AI solutions. Therefore, having a clear strategy for successful integration is essential.
A structured approach should begin with evaluating the current technological landscape and identifying areas where AI can add the most value. This assessment can guide organizations in prioritizing AI projects that align with their business objectives. Ensuring alignment between AI initiatives and overall business strategies can enhance the efficacy of integration.
Next, organizations should pilot AI projects with manageable scopes to test interoperability with existing systems. Engaging stakeholders throughout the process allows for feedback and adjustments, ensuring technology fits into the organizational culture. Moreover, fostering collaboration between IT teams and business units is critical in overcoming integration barriers.
7. Scalability
The deployment of AI solutions often leads to increased demand as organizations recognize their value. However, scalability poses a significant challenge; AI systems must be capable of effectively managing growing volumes of data without sacrificing performance.
Robust infrastructure is a key element in ensuring scalability. Organizations should invest in cloud technologies that can dynamically allocate resources based on demand. This flexibility enables AI solutions to handle fluctuations in usage while maintaining processing speeds.
Additionally, continuous monitoring is vital in identifying potential performance bottlenecks. Implementing feedback loops can help organizations fine-tune their AI models over time, ensuring they remain effective at scale. The cultivation of a culture centered on experimentation and continuous improvement can further enhance long-term scalability.
8. Talent Acquisition and Retention
The demand for skilled AI professionals has surged, creating a talent shortage that poses a substantial challenge for organizations. Recruiting and retaining top talent is essential to foster innovation and maintain a competitive edge in the AI landscape.
Organizations should consider investing in training programs that empower existing employees with AI knowledge and skills. Offering upskilling opportunities can boost employee morale and reduce turnover while creating a more competent workforce.
Moreover, creating an attractive work environment is pivotal in attracting talent. Emphasizing aspects such as work-life balance, opportunities for professional growth, and fostering a diverse and inclusive workplace culture can enhance recruitment efforts. Collaborating with universities and research institutions can also provide a pipeline of talent and fresh ideas.
9. Ethical Considerations
The responsible deployment of AI technologies requires addressing ethical issues head-on. Ethics in AI encompasses concerns about ensuring that systems respect individual rights, do not perpetuate discrimination, and are developed transparently.
Organizations should establish ethical guidelines for AI development that reflect their values and societal obligations. This includes creating ethical review boards to evaluate the implications of AI projects before implementation. Engaging stakeholders in discussions about ethical considerations can produce better outcomes and foster accountability.
Implementing privacy-by-design principles, where ethical considerations are integrated from the inception of AI projects, can significantly enhance ethical compliance. Continuous evaluation and adjustment based on ethical standards create a culture of responsibility that nurtures trust among users.
10. Change Management
Successful AI implementation is often hindered by change management challenges. Employees may resist adopting new technologies due to fear of job displacement or lack of understanding of AI’s benefits.
Organizations should prioritize comprehensive change management programs that educate employees on how AI can augment their roles rather than replace them. Providing regular updates about the progress and successes of AI initiatives can help alleviate concerns about job security.
Fostering a culture that embraces innovation is also essential. Organizations can encourage experimentation and exposure to AI technologies through workshops, training, and pilot programs. By empowering employees to take part in the AI journey, organizations can cultivate a sense of ownership that facilitates smoother transitions.
By actively confronting these ten new AI challenges, organizations can better harness the transformative potential of artificial intelligence. Proactively addressing these hurdles sharpens their AI capabilities, enables organizations to meet market demands, and maintains a competitive edge. For more insights on leveraging AI effectively, visit AIwithChris.com today.
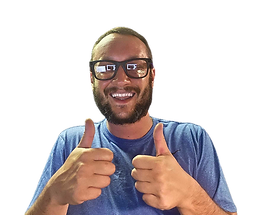_edited.png)
🔥 Ready to dive into AI and automation? Start learning today at AIwithChris.com! 🚀Join my community for FREE and get access to exclusive AI tools and learning modules – let's unlock the power of AI together!